Note
Go to the end to download the full example code.
Compare LogisticRegression solver with sklearn’s liblinear backend¶
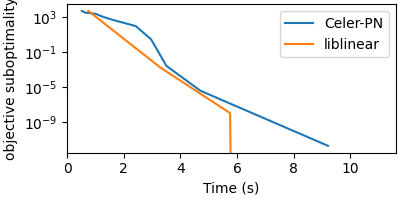
import time
import warnings
import numpy as np
from numpy.linalg import norm
import matplotlib.pyplot as plt
from sklearn import linear_model
from libsvmdata import fetch_libsvm
from celer import LogisticRegression
warnings.filterwarnings("ignore", message="Objective did not converge")
warnings.filterwarnings("ignore", message="Liblinear failed to converge")
X, y = fetch_libsvm("news20.binary")
C_min = 2 / norm(X.T @ y, ord=np.inf)
C = 20 * C_min
def pobj_logreg(w):
return np.sum(np.log(1 + np.exp(-y * (X @ w)))) + 1. / C * norm(w, ord=1)
pobj_celer = []
t_celer = []
for n_iter in range(10):
t0 = time.time()
clf = LogisticRegression(
C=C, solver="celer-pn", max_iter=n_iter, tol=0).fit(X, y)
t_celer.append(time.time() - t0)
w_celer = clf.coef_.ravel()
pobj_celer.append(pobj_logreg(w_celer))
pobj_celer = np.array(pobj_celer)
pobj_libl = []
t_libl = []
for n_iter in np.arange(0, 50, 10):
t0 = time.time()
clf = linear_model.LogisticRegression(
C=C, solver="liblinear", penalty='l1', fit_intercept=False,
max_iter=n_iter, random_state=0, tol=1e-10).fit(X, y)
t_libl.append(time.time() - t0)
w_libl = clf.coef_.ravel()
pobj_libl.append(pobj_logreg(w_libl))
pobj_libl = np.array(pobj_libl)
p_star = min(pobj_celer.min(), pobj_libl.min())
plt.close("all")
fig = plt.figure(figsize=(4, 2), constrained_layout=True)
plt.semilogy(t_celer, pobj_celer - p_star, label="Celer-PN")
plt.semilogy(t_libl, pobj_libl - p_star, label="liblinear")
plt.legend()
plt.xlabel("Time (s)")
plt.ylabel("objective suboptimality")
plt.show(block=False)
Total running time of the script: (1 minutes 13.707 seconds)